Introduction:
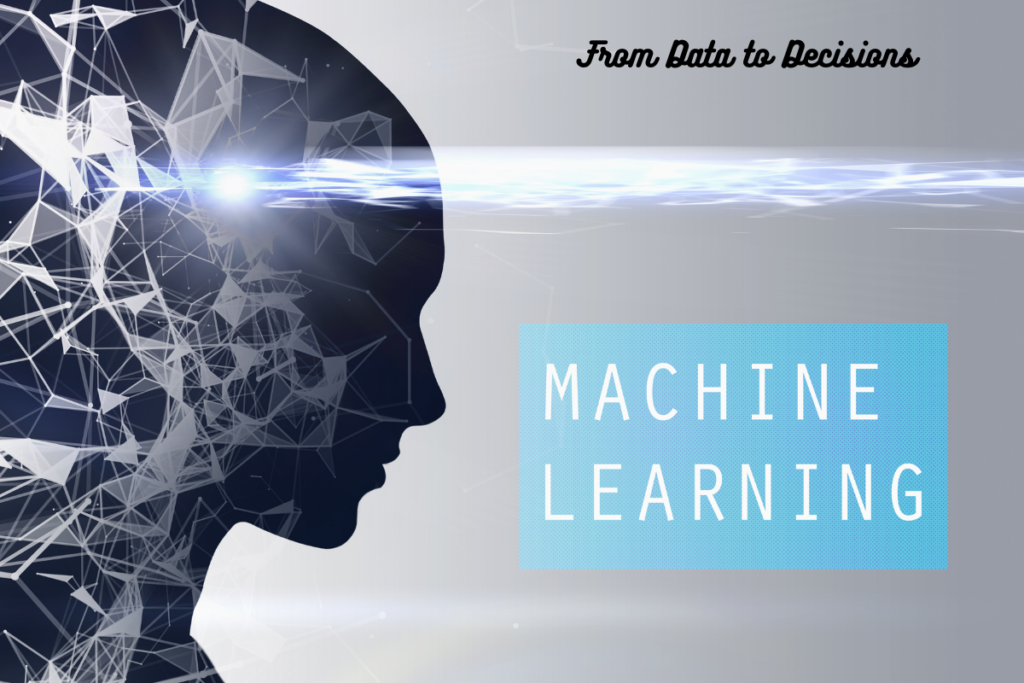
“From Data to Decisions: The Power of Machine Learning” shows how machine learning transforms data interpretation and action. Information is abundant and complex in today’s data-driven society. However, machine learning can find patterns and insights in massive datasets that people cannot. Effective data analysis helps firms make educated decisions, improve operational efficiency, and improve consumer experiences.
The concept of “From Data to Decisions,” which emphasizes how raw data becomes meaningful, underpins this method. Machine learning algorithms improve their accuracy and relevance to specific challenges from existing data. This lets companies respond to trends and foresee future events, driving strategic initiatives. Machine learning helps firms smoothly go from data collection to actionable insights, driving innovation and competitive advantage.
Table of Contents
Data Collection:
Effective decision-making in today’s data-driven world requires methodical data collection from varied sources. Organisations now have unparalleled data on consumer interactions, market trends, and operational metrics. Businesses must first obtain relevant and accurate data to use this data. Data quality and depth affect the efficiency of following analysis and insights, making this phase critical. In this early step, “From Data to Decisions” takes shape.
In “From Data to Decisions,” data collecting is crucial. Surveys, internet tracking, and sensor inputs can help organizations create a multivariate dataset. This vast dataset feeds machine learning algorithms, which examine it to gain insights. The purpose is to collect massive, diverse, and relevant data to help the company make decisions by drawing meaningful connections and conclusions.
Effective data collection tactics can also improve decision-making efficiency. Businesses build a historical record of data collection and storage that reveals trends and patterns. A solid data foundation helps firms make informed “From Data to Decisions” decisions. Understanding all available data helps firms make strategic decisions that accomplish their goals, respond to market changes, and satisfy customers. Thus, data collecting is not simply a preliminary step but an essential aspect of the decision-making framework that can lead to long-term success.
Data Processing:
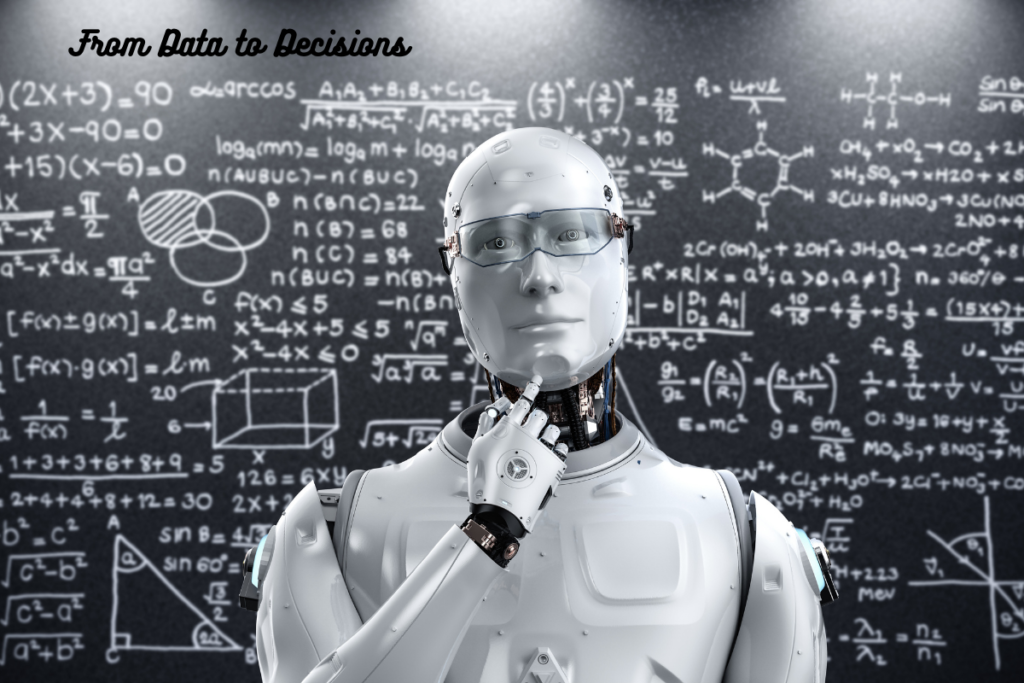
Data processing is essential to decision-making after data collection. To enable accurate analysis, this phase cleans and preprocesses data. Data formats vary and may contain errors or missing values. Therefore, companies must use rigorous data cleaning methods to improve dataset quality and reliability. Foundational work is crucial since it affects later insights’ accuracy. Businesses improve their “From Data to Decisions.” transition by formatting raw data.
In “From Data to Decisions” context, data pretreatment for clarity and relevance is crucial. This stage uses normalization, standardization, and transformation to prepare data for machine learning algorithms. Delete duplicates, fill in missing values, and filter out extraneous information are crucial duties in this phase. Well-processed datasets improve analytical accuracy and decision-making speed. Well-organized and cohesive data helps decision-makers spot patterns and trends.
Effective data processing helps firms gain deeper insights and make more complex decisions. As they use “From Data to Decisions,” firms seek both instant answers and the underlying causes. High-quality, processed data enables richer analysis, allowing firms to foresee trends, identify difficulties, and exploit opportunities. In conclusion, accurate data processing underpins informed decision-making and strategic objectives. “Living with AI: Machine Learning impact day-to-day activities” is becoming increasingly important in this process, as AI-powered tools help analyze data and uncover insights that would otherwise be hidden.
Pattern Recognition:
Pattern recognition is crucial to “From Data to Decisions,” especially in machine learning algorithms. After data gathering and processing, advanced approaches are used to find hidden patterns and correlations. These algorithms swiftly and efficiently evaluate massive volumes of data to find relationships that humans may miss. Organizations can change their strategy by identifying these data subtleties.
Through “From Data to Decisions,” pattern recognition lets organizations go beyond data analysis. While traditional methods highlight fundamental indicators, machine learning allows deeper data examination. Algorithms can disclose client purchase habits, market trends, and operational inefficiencies, improving company understanding. This capacity lets decision-makers consider many performance-affecting aspects. Organizations can gain actionable insights for informed decision-making by using advanced pattern recognition.
Pattern recognition helps predictive analytics forecast future outcomes based on historical data. As firms go “From Data to Decisions,” predicting trends, customer behavior, and dangers is crucial. For instance, customer data trends can inform marketing, product development, and inventory management, helping companies stay ahead of the competition. Pattern identification with machine learning connects raw data to strategic decisions, making data a valuable tool for innovation, efficiency, and growth.
Predictive Analytics:

Predictive analytics transforms organizations by using historical data to predict future patterns and behaviors. Businesses can use statistical algorithms and machine learning to evaluate past occurrences and find patterns to anticipate future events. Organisations need this competence to make proactive rather than reactive decisions. Predictive analytics helps firms understand their operations and improve strategic planning.
In “From Data to Decisions,” predictive analytics helps firms design their plans. Companies can better allocate resources by evaluating data patterns to discover opportunities and threats. Seasonal tendencies can help shops estimate inventory demands, while previous borrower behavior can help financial institutions analyze credit risk. Through historical data analysis, this foresight bridges the gap between data observation and informed decision-making, allowing businesses to create evidence-based strategies.
When organizations use predictive analytics, the “From Data to Decisions” transition is substantially improved. Companies may make data-driven, long-term decisions by anticipating market trends, client preferences, and operational issues. They can boost innovation, consumer satisfaction, and performance. As firms integrate predictive analytics into their decision-making processes, they turn raw data into actionable insights, improving their ability to navigate modern business.
Decision Support Systems:
Modern enterprises need Decision Support Systems (DSS) to analyze data and provide actionable insights. These systems use machine learning to make evidence-based strategic recommendations, changing business strategy. DSS can collect massive amounts of data and help firms find the most relevant insights using sophisticated algorithms and data analysis. The journey “From Data to Decisions” becomes more structured and efficient when decision-makers have accurate information.
In “From Data to Decisions,” machine learning improves Decision Support Systems by enabling real-time scenario analysis and modeling. These systems may simulate outcomes based on multiple decision pathways using historical data, allowing organizations to assess their options before implementation. This is especially useful in dynamic contexts where quick, informed decisions are crucial for competitiveness. Therefore, firms may reduce risks and maximize opportunities by using data-backed insights instead of intuition.
Machine learning in Decision Support Systems encourages collaboration in decision-making. Data analysis simplifies decision-making, allowing stakeholders across departments to debate evidence-based issues. This collaborative approach strengthens “From Data to Decisions” and promotes organizational transparency and responsibility. Businesses can increase strategy alignment and performance by using well-supported data rather than assumptions, leading to better results in uncertain times.
Continuous Learning:

Machine learning is based on continuous learning, which helps businesses make dynamic and adaptive decisions. “Machine learning algorithms” thrive on iterative data analysis, where new data informs and refines ideas. This allows firms to improve their tactics to match the ever-changing industry situation. Continuous learning helps firms adapt to new trends, problems, and opportunities via the “From Data to Decisions” method.
In “From Data to Decisions,” continual learning keeps data analysis insights relevant and correct. Machine learning models enhance their forecasts and recommendations with fresh data, giving enterprises a better decision-making base. This implies businesses are using both historical and current data to inform their plans. Organizations must respond quickly to changes in customer behavior, market conditions, and operational disturbances to stay competitive.
Continuous learning also encourages innovation and growth in enterprises. Companies can try new things with minimal risk by using machine learning’s iterative capabilities. The move “From Data to Decisions” forms a feedback loop, improving future decisions with new data. This strategy refines existing strategies and supports new solutions and procedures to accomplish company goals. Continuous machine learning enables a more robust and forward-thinking decision-making framework, enabling sustained success in a quickly changing world.
Real-time Insights:
Today’s fast-paced corporate climate requires quick and informed decisions, and real-time insights enable this. Machine learning lets firms examine real-time data and respond quickly to changing conditions. Financial, healthcare, and e-commerce industries need this expertise for timeliness. Real-time insights change “From Data to Decisions,” enabling firms to be proactive in meeting operational and market needs.
In the “From Data to Decisions” paradigm, real-time insights improve situational awareness. Machine learning algorithms can detect trends, abnormalities, and patterns in streaming data. This instantaneous feedback loop lets decision-makers optimize outcomes and minimize risks quickly. Real-time analytics can predict supply chain delays and recommend alternative routes, and they can adjust marketing incentives to customer behavior. Timing interventions help firms meet strategic goals in a changing market.
Real-time insights also encourage ongoing development and innovation, which is crucial for long-term success. Companies get a competitive advantage by swiftly iterating on their plans and operations when they use real-time analysis in their decision-making. Businesses use the latest data to increase productivity, service delivery, and customer satisfaction, making the “From Data to Decisions” transfer smooth. This competence helps firms stay ahead of the competition and react rapidly to unexpected obstacles, ensuring resilience and sustainability in an unpredictable business world. “AI in Education: Personalized Learning, Adaptive Tutoring and Democratizing access to Education” exemplifies this dynamic. AI is transforming education by providing personalized learning experiences, adaptive tutoring systems, and greater accessibility to educational resources.
Enhanced Customer Experiences:

In a competitive market, businesses must improve consumer experiences to generate loyalty and prosper. Companies may adjust products, services, and interactions to target audiences’ needs and expectations by knowing customer data and preferences. This approach analyzes massive customer data with machine learning to find insights that inform more tailored and effective initiatives. The capacity to turn raw data into actionable insights that meet client needs enhances “From Data to Decisions”.
Leveraging consumer data allows firms to personalize experiences under the “From Data to Decisions” framework. Machine learning algorithms can segment customers by behavior, preferences, and comments, helping companies create focused marketing campaigns, new product features, and better customer support. Personalization improves consumer engagement and satisfaction. E-commerce sites can promote things based on browsing history and past transactions, while streaming services can recommend material based on viewing patterns.
Data-driven customer experience improvements strengthen consumer relationships and brand loyalty. Businesses may better anticipate consumer requirements and address challenges when they use customer data in their decision-making. This proactive strategy builds brand trust and appreciation by making customers feel understood and valued. When firms go “From Data to Decisions,” they can quickly adjust to changing customer expectations and industry trends, producing a customer-centric business model that drives long-term profitability and market uniqueness. “AI and the Future of Work: How Artificial Intelligence change the way we work” is a significant aspect of this transformation. AI is changing the nature of work, automating tasks, creating new jobs, and requiring workers to adapt to new skills and technologies.
Operational Efficiency:
Organizations seeking to optimize production and eliminate waste and increase profitability must prioritize operational efficiency. Businesses may optimize operations by using data analysis to understand their processes and resource use. This technique relies on machine learning to discover inefficiencies, estimate demand, and offer data-driven solutions. As firms move from “From Data to Decisions,” they gain the skills to reduce workflows and boost efficiency across their operations.
Data analysis helps firms maximize resource allocation in the “From Data to Decisions” paradigm. Companies may better understand their supply chains, manufacturing schedules, and staff management by analyzing historical and real-time data. This knowledge helps companies allocate resources, decreasing downtime and bottlenecks. Machine learning-powered predictive maintenance can foresee equipment breakdowns, allocating resources to prevent interruptions rather than respond to them. Therefore, enterprises may retain continuity and increase service delivery without overspending.
Data-driven process optimization promotes innovation and ongoing improvement, which are vital for modern business competitiveness. Based on reliable data analysis, firms enhance their operational strategy and develop an efficient culture throughout the organization. This transition “From Data to Decisions” improves procedures and fosters the development of new methods and technologies to boost efficiency. Data insights help firms adapt and evolve to meet future problems and capture opportunities, assuring industry success and growth.
Risk Management:
Effective risk management is essential to modern enterprises’ strategic planning in an uncertain world. Businesses can reduce risks and develop resilience by identifying and assessing risks. Data analysis and machine learning help firms anticipate vulnerabilities and prepare, turning raw data into usable insight. As businesses go from “From Data to Decisions,” they use insights to secure operations and seize risk landscape opportunities.
In “From Data to Decisions,” data-driven risk management monitors and analyzes internal and external elements that potentially affect business performance. Market trends, economic indicators, and operational metrics can be integrated into machine learning models to forecast and analyze strategic decisions. This competence lets businesses anticipate hazards and create contingency plans before interruptions. Financial institutions can use predictive analytics to manage credit risk and preserve financial stability, while supply chain managers can foresee logistical bottlenecks and alter procurement strategy.
Effective risk management boosts an organization’s resilience and adaptability, essential for staying ahead in a changing world. Companies foster agility and preparation by integrating risk assessment into “From Data to Decisions”. This proactive approach reduces losses and helps firms to innovate and pursue strategic goals. Businesses strengthen their risk management procedures to ensure long-term performance and resilience in the face of challenges and market disruptions.
Conclusion:
In conclusion, “From Data to Decisions: The Power of Machine Learning” shows how data-driven insights may alter corporate decision-making. Businesses can make evidence-based, foresight-based decisions by gathering and processing data, finding patterns, and using predictive analytics. Machine learning’s continuous learning and real-time insights improve these processes, keeping firms nimble and responsive to new problems and opportunities. This “From Data to Decisions” journey helps firms integrate strategic efforts with data-backed insights, improving efficiency, innovation, and competitiveness.
Beyond operational gains, machine learning in decision-making transforms how organizations think about and solve problems. Intelligent data analysis improves customer experiences, operational efficiency, risk management, and decision support systems, creating a proactive and forward-thinking organisation. Businesses who embrace the move “From Data to Decisions,” increase their ability to adapt and succeed in a fast changing business landscape, setting the framework for digital era development and success
People Also Ask:
Generative AI models today have trouble with factual accuracy, biases in training data, and coming up with truly new material. Researchers are working to fix these problems by filtering data better, finding ways to reduce bias, and making training datasets that are more varied.
Building stable quantum computers and making programs that fully use their unique features are hard for quantum machine learning. But there are chances to change the way drug development, materials science, and optimization problems are solved, which could lead to big steps forward in many fields.
Pingback: The Impact of Machine Learning on Finance
Pingback: The Future of Machine Learning: What Expect in Next Decade